Grants Funded
Grant applicants for the 2024 cycle requested a total of nearly $3 million dollars. The PSF Study Section Subcommittees of Basic & Translational Research and Clinical Research evaluated more than 100 grant applications on the following topics:
The PSF awarded research grants totaling over $650,000 dollars to support more than 20 plastic surgery research proposals.
ASPS/PSF leadership is committed to continuing to provide high levels of investigator-initiated research support to ensure that plastic surgeons have the needed research resources to be pioneers and innovators in advancing the practice of medicine.
Research Abstracts
Search The PSF database to have easy access to full-text grant abstracts from past PSF-funded research projects 2003 to present. All abstracts are the work of the Principal Investigators and were retrieved from their PSF grant applications. Several different filters may be applied to locate abstracts specific to a particular focus area or PSF funding mechanism.
Predicting Breast Reconstruction Patient Reported Outcomes with Machine Learning
Jonlin Chen MD
2024
Memorial Sloan Kettering Cancer Center (MSK)
Research Fellowship
Breast (Cosmetic/Reconstructive), Technology Based
Project Summary: In breast reconstruction patients, patient-reported outcomes (PROs) are particularly important because the goal of reconstruction is to ultimately enhance patient well-being and satisfaction. However, existing surgical outcomes prediction models in breast cancer patients have fallen short because they are limited to a small number of variables and cannot account for elaborate higher-order functions or nonlinear relationships between variables. This hinders the accurate prediction of individualized PROs in breast reconstruction patients and prevents providers from using this data to inform surgical decision-making. Machine learning (ML) has recently emerged as a possible solution to this problem. Our preliminary studies have demonstrated three validated ML algorithms to predict individualized PROs at 2-year follow-up for women with breast cancer. Thus, the goal of this study is to optimize machine learning algorithms to better predict post-operative PROs and produce a clinical decision-making tool to aid in individualized risk assessment, preoperative counseling, and expectation management. We will do this by systematically investigating the performance of ML algorithms to ensure optimal algorithm selection. Optimized algorithms will be incorporated into a mobile application that guides the reconstructive surgeon through patient-specific inputs and presents an individualized, pre-operative prediction of PROs per breast reconstruction option (e.g., autologous reconstruction vs. implant-based reconstruction).
Impact Statement: This project holds transformative potential in breast reconstruction. By using machine learning, we can more accurately predict individualized PROs for breast reconstruction patients. The resulting mobile app will be a catalyst for the clinical use of our algorithm for personalized risk assessment and better-informed preoperative counseling. This innovation pushes forward a broader trend in healthcare towards data-driven precision. The ultimate impact of this proposed project extends beyond breast reconstruction by setting a precedent for the integration of artificial intelligence into clinical practice to improve the patient experience.
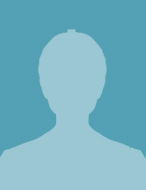