Grants Funded
Grant applicants for the 2024 cycle requested a total of nearly $3 million dollars. The PSF Study Section Subcommittees of Basic & Translational Research and Clinical Research evaluated more than 100 grant applications on the following topics:
The PSF awarded research grants totaling over $650,000 dollars to support more than 20 plastic surgery research proposals.
ASPS/PSF leadership is committed to continuing to provide high levels of investigator-initiated research support to ensure that plastic surgeons have the needed research resources to be pioneers and innovators in advancing the practice of medicine.
Research Abstracts
Search The PSF database to have easy access to full-text grant abstracts from past PSF-funded research projects 2003 to present. All abstracts are the work of the Principal Investigators and were retrieved from their PSF grant applications. Several different filters may be applied to locate abstracts specific to a particular focus area or PSF funding mechanism.
Improving Outcome Prediction in Breast Reconstruction Using Machine Learning
Jonas Nelson MD
2023
University of Texas M.D. Anderson Cancer Center
National Endowment for Plastic Surgery Grant
Breast (Cosmetic/Reconstructive), Technology Based
Project Summary: Complications occur commonly after breast reconstruction resulting in patient distress, suboptimal aesthetic outcomes, higher health care costs, and delays in oncologic treatment. Previous studies have developed nomograms to predict surgical risks based on large databases. Although these studies have been helpful and can help guide clinical care, current risk prediction models rely on the use of logistic regression that may over or underestimate risks for some patients. Machine learning (ML), a subset of artificial intelligence, involves developing and employing algorithms capable of identifying correlations and patterns of complex risk factors regardless of statistical significance. Using non-linear techniques, ML can "learn" patterns between complex variables and help develop more accurate models to predict outcomes after breast reconstruction. In preliminary studies, we have used ML to develop robust, comprehensive, integrated predictive models using our institutional database. These models demonstrated higher performance compared with previously reported logistic regression nomograms. In addition, these models recognized the non-linear multifactorial nature of adverse events. The purpose of this proposal is to externally validate our models using additional data and compare their performance to previously reported logistic regression models. ML models will identify patients who are at high risk of developing complications using non-linear predictors and help guide surgical planning and patient education. Our central hypothesis is that ML models provide superior outcomes prediction for breast reconstruction patients compared to available statistical models. The rationale is that use of logistic regression in current predictive models limits their effectiveness, predictive power, and generalizability. Our approach is distinguished by the use of AI-driven predictive models that overcome traditional risk prediction barriers by incorporating non-linear algorithms capable of accurately predicting clinical outcomes. We will test our central hypothesis and achieve our objective with the following specific aims: Aim 1: Externally validate and augment ML predictive models for breast reconstruction outcomes. Aim 2: Compare the performance of ML models to current risk assessment tools models in predicting breast reconstruction outcomes.
Impact Statement: This proposal will deliver artificial intelligence predictive models to identify patients who are at high risk of developing complications using non-linear predictors and help guide surgical planning and patient education. Thereby these ML models will significantly improve breast reconstruction outcomes; resulting in a significant advancement and positive impact for breast reconstruction. The product of this project will be used in a future proposal for federal funding to integrate these models into a clinical decision support-mobile user interface and assess their impact in a before-after, prospective observational study evaluating baseline practice patterns, patient and surgeon satisfaction with care, use of preoperative optimization, changes in surgical planning, and complication rates.
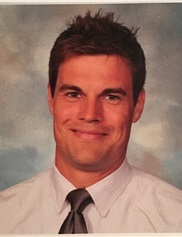