Grants Funded
Grant applicants for the 2024 cycle requested a total of nearly $3 million dollars. The PSF Study Section Subcommittees of Basic & Translational Research and Clinical Research evaluated more than 100 grant applications on the following topics:
The PSF awarded research grants totaling over $650,000 dollars to support more than 20 plastic surgery research proposals.
ASPS/PSF leadership is committed to continuing to provide high levels of investigator-initiated research support to ensure that plastic surgeons have the needed research resources to be pioneers and innovators in advancing the practice of medicine.
Research Abstracts
Search The PSF database to have easy access to full-text grant abstracts from past PSF-funded research projects 2003 to present. All abstracts are the work of the Principal Investigators and were retrieved from their PSF grant applications. Several different filters may be applied to locate abstracts specific to a particular focus area or PSF funding mechanism.
Artificial Intelligence for Diagnosing Occult Scaphoid Fractures in Radiographs
Alfred Yoon MD
2020
University of Michigan, Section of Plastic Surgery
National Endowment for Plastic Surgery Grant
Technology Based, Hand or Upper Extremity
Project Summary: Scaphoid fracture is the most common carpal bone fracture in adults with the potential for life-long quality-of-life and functional decline. Some scaphoid fractures are not visible to humans in the initial injury radiograph, which presents a critical diagnostic challenge to physicians. Because of such occult fractures, the conventional treatment for suspected scaphoid injury is empiric immobilization in a cast and serial follow-up X-rays for up to 6 weeks. Such practice comes at a substantial cost to the patient from lost productivity, sacrifice in quality of life, and time spent traveling to clinic visits. Advanced imaging such as computed tomography (CT) scans and magnetic resonance imaging (MRI) can be used to diagnose occult scaphoid fractures. But administering advanced imaging to all patients with possible occult scaphoid fractures will increase healthcare expenditure considerably and expose patients to unnecessary radiation. In this study, we will use scaphoid radiographs from Michigan Medicine and Chang Gung Memorial Hospital to study three aims: Aim 1 will create a convolutional neural network based on artificial intelligence that can detect occult scaphoid fractures in the initial injury radiograph, Aim 2 will assess the efficacy of the convolutional neural network as a clinical adjunct tool, and Aim 3 will determine the cost-effectiveness of diagnosing occult scaphoid fractures using the convolutional neural network compared to conventional methods. This study is novel in the use of heterogeneous radiographic databases from two institutions and apply artificial intelligence to create a robust convolutional neural network that may change scaphoid fracture management. The results of this study will improve healthcare delivery by preventing morbidity associated with missed occult scaphoid fractures and provide a cost-effective method to detect occult scaphoid fractures in a timely fashion to avoid unnecessary burdensome casting. Impact Statement: Scaphoid fracture is the most common wrist bone injury, which when left untreated can result in irreversible bony damage that may lead to chronic pain and lost work potential. Unfortunately, a subset of scaphoid fractures are occult fractures that are not visible on the initial injury X-rays. Therefore, in patients for whom such occult fractures are suspected, they are either managed with precautionary casting for 6 weeks or undergo expensive imaging such as CT scans or MRI. We aim to utilize the recent advances in artificial intelligence to develop a model that can detect occult scaphoid fractures in the initial X-rays. The early detection of occult scaphoid fractures will decrease time to definitive management, alleviate patients' financial burden, and increase patients' quality of life.
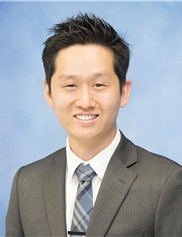